How translation apps iron out embarrassing gaffes
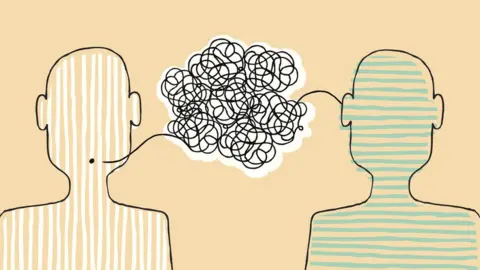
Translation apps are getting better, but they're still not perfect, particularly for minority languages. Can artificial intelligence and deep neural networks help iron out the glitches?
During the World Cup in Russia this summer there was a dramatic spike in the use of Google Translate, the company says, as fans tried to strike up conversations with their hosts and fellow fans from around the world.
The words for "stadium" and "beer" were in particularly high demand.
These days the traditional phrasebook is on the way out. A recent survey from the British Council found that nearly two-thirds of 16 to 34-year-olds now rely on translation apps to help navigate the local lingo.
But while such apps are undoubtedly getting better, they're still not totally reliable - a fifth of those surveyed said they experienced misunderstandings while on holiday because of mistranslations on their phone.
The issue is particularly acute for speakers of non-mainstream languages.
Welsh people, for example, have been noticing some particularly "scummy" translations. One warning sign reading "Blasting in Progress" was rendered as "Gweithwyr yn ffrwydro" or "workers exploding", for example.
And this summer, a Google Translate user discovered that typing "dog" 18 times produced a Maori translation reading: "Doomsday Clock is three minutes at twelve We are experiencing characters and a dramatic developments in the world, which indicate that we are increasingly approaching the end times and Jesus' return."
So why are translations glitches still happening in the age of supercomputers and machine learning?
One big problem is that words often have more than one meaning. These homographs, as they're called, can lead to embarrassment not just for holidaymakers but for governments as well.
Take the UK government's botched German version of the Brexit white paper in July, which translated the phrase "democratic exercise" as "demokratische Übung" - where "Übung" meant physical exercise not practice.
To deal with mistakes like this, translation apps are continuously refining the ways in which machine learning is applied. They make use of previously translated texts to provide their answers, checking the context in which the word has been used before and selecting the most likely meaning.
Earlier this year, Microsoft announced that it had achieved "human parity" in the quality of its translations. A set of Chinese news articles were machine translated into English and a team of independent experts found that they were on a par with translations provided by two professional translators.
The key to this breakthrough was the use of deep neural networks, Microsoft said, as well as statistical machine translation.
Simply put, this involved refining the first "rough" translation by going back over the results several times in each direction, comparing and contrasting and learning each time, in a similar way to a human.
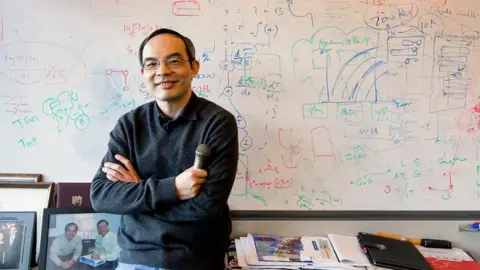
A translation system already has a fair idea of what a grammatical sentence in each language looks like based on all the documents it's learned from in the past.
"Rather than writing handcrafted rules to translate between languages, modern translation systems approach translation as a problem of learning the transformation of text between languages from existing human translations and leveraging recent advances in applied statistics and machine learning," explains Xuedong Huang, technical fellow, speech and language, at Microsoft Research.
Reaching human parity sounds like a pretty impressive achievement. But even Microsoft admits that translating historic news articles is not the same as translating live human conversation, where the nuances of idiom, accent and dialect present a much bigger challenge.
Last year, Google launched wireless in-ear headphones called Pixel Buds that can translate 40 languages in real-time - although how accurately it can do this is up for debate. And New York-based start-up Waverly Labs has developed its own Pilot Translating Earpiece and smartphone app that can translate 15 languages in near real-time, the company says.
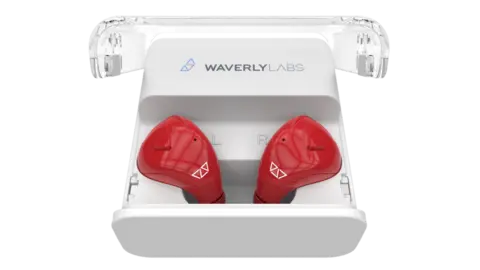
But when you're trying to translate between two languages for which there isn't such a broad database of translated documents to learn from - Sinhala to Pashto, for example - the challenge is all the greater.
It's possible to produce a translation of sorts by translating Sinhala to English and then translating the result into Pashto, but this clearly introduces errors of the type already mentioned above.
In the case of the apocalyptic rendering of multiple Maori dogs, one reason for the strange result is that for rarer languages, there's an over-reliance on documents that do exist in both languages: in this case, the Bible.
"If you train your model with parallel sentences coming from an old manuscript, and try to translate a conversation between people talking nowadays, the model will be very confused because both the content and the style of today's conversations will be very different from what you will find in the manuscript," says Facebook AI researcher Guillaume Lample.
"Also, the model is likely to generate segments of words that it found in that manuscript. This kind of issue is something that is likely to happen on low-resource languages for which the amount of parallel sentences is very small, and where old documents will represent a significant amount of the overall parallel data."

More Technology of Business

But a new project from Mr Lample and a team of other researchers at Facebook and the Sorbonne University in Paris may represent a way round this problem.
They are using source texts of just a few hundred thousand sentences in each language, but no directly translated sentences at all.
Essentially, the team's system looks at the patterns in which words are used. For example, the English words "cat" and "furry" tend to appear in a similar relationship as "gato" and "peludo" in Spanish. The system learns these so-called word embeddings, allowing it to infer a "fairly accurate" bilingual dictionary.
It then applies the same back-and-forth techniques as we've seen with Microsoft Translator to come up with its final translation - and not a biblical reference in sight.
In fact, says Mr Lample, the pattern-mapping technique could potentially have applications beyond currently used languages - deciphering lost ones, for example.
"There is a major obstacle though, which is the amount of sentences we can gather in these languages. For instance, the Voynich manuscript (a 15th Century codex that has defied translation so far) only contains a few hundred pages of text, which is too small for our model to work," he says.
"But if we were able to gather a reasonable amount of text, we should be able to revive dead languages."
And there may even be more exciting possibilities further afield.
"We may be able to learn to communicate with friendly aliens," Mr Lample suggests. "But first they would need to talk a lot - and about things relatively similar to what we talk about among ourselves."
A case of "Lost in Translation" meets "Lost in Space" perhaps?